Organiser: Loes Ottink (Noldus Information Technology, The Netherlands) and Lucas Noldus (Radboud University, Nijmegen, The Netherlands and Noldus Information Technology, The Netherlands).
Schedule: Friday 17th, 10:00 – 12:10 Meeting Room 7
10:00 – 10:20 Liezl Maree – Multi-view triangulation-enabled annotation for multi-animal 3D pose in SLEAP
Deep learning markerless motion capture via SLEAP (Social LEAP Estimates Animal Poses) enables high-resolution behavioral kinematics measurement for multiple animals, ideal for social behavior studies. However, close-proximity social interactions often cause occlusions, impacting tracking performance. We address this with multi-camera solutions, expanding SLEAP’s 2D pose estimation toward ongoing development of 3D pose estimation capabilities. In this paper, we propose practical multi-view approaches and outline a pipeline for multi-animal 3D pose tracking.
10: 20 – 10:40 Caleb Weinreb – Parsing the sub-second structure of animal behavior with Keypoint-MoSeq
We present keypoint-MoSeq, a machine learning-based platform for unsupervised learning of behavioral modules (“syllables”) from animal pose tracking. Keypoint-MoSeq outperforms alternative clustering methods at identifying kinematc transitions, at capturing correlations between neural activity and behavior, and at classifying either solitary or social behaviors in accordance with human annotations. Keypoint-MoSeq also generalizes across species and timescales, identifying a spectrum of oscillatory behaviors in fruit flies.
10:40 – 11:00 Timon Daniels – Fast annotation of Rodent Behaviors with AI Assistance: Human Observer and Smart Annotator collaborate through Active Learning
AI-assisted behavior annotation saves time compared to manual annotation. Although automated systems for rodent behavior annotation exist, specific behaviors are still scored by hand. With active learning, we can reduce the annotation effort and tailor the result towards the needs within a research experiment. The results are not equally accurate over behaviors. We present a case of annotating ‘stretched attend’ that benefits from a cascading solution that zooms in on a subset of the data.
BREAK
11:30 – 11:50
Adrian Loy – DeepRod: A human-in-the-loop system for automatic rodent behavior analysis.
We present a human-in-the-loop system for efficient rodent behavior analysis in drug development. Addressing the time-consuming and labor-intensive nature of manual behavior categorization, this UX-optimized platform integrates AI for complex behavior prediction and active learning to identify rare events. The proposed solution leverages a cloud-native data processing pipeline, AI-based novelty behavior recognition and multi-class classification, demonstrating significant improvements in behavior labeling and discovery.
11:50 – 12:10 Vivek Kumar – End-to-end behavior annotation pipeline for mouse behavior annotation.
Developments in computer-vision have greatly advanced automated behavior annotation. An integrated hardware and software solution is necessary to facilitate the adoption of these advances, particularly for non-computational labs. Here, we present our integrated rodent phenotyping platform, JAX Animal Behavior System (JABS), to the community for data acquisition, machine learning-based behavior annotation and classification, classifier sharing, and genetic analysis. This open-source tool is an ecosystem that reduces the barrier to entry into this new field.
Description:
As advances in AI development and deployment are rapidly evolving, the implementations in behavioral neuroscience are increasing as well. Behavioral research in laboratory animals such as rodents, flies and zebrafish has benefited a lot from developments in machine learning and computer vision algorithms and models and their performance. Accurate analysis of behavior is important in research areas such as neuroscience, psychology and pharmacology. It is essential in for instance preclinical research to investigate treatment efficacy in animal models for diseases and disorders, and for other advancements in biomedical and genetic research. Nevertheless, robust video tracking, pose estimation and automatic recognition of complex behaviors, especially in group settings, still remain challenges that the field is confronted with.
Robust video tracking and pose estimation are prerequisites for accurate behavior recognition in laboratory animals, especially in social and other complex behaviors, and in semi-natural environments or cages with occluding objects. Deep learning algorithms and computer vision are being deployed for body point detection, pose estimation and tracking over time, and subsequently, AI models are trained to recognize behaviors from this data. Automating tracking and behavior recognition reduces the role of human labeling of behavior in videos, making it less time-consuming. Furthermore, it decreases variation between researchers and in annotations of ambiguous behaviors, leading to more reliable results. These methods face challenges when involving for instance semi-natural environments or situations with a lot of social interaction. However, with the rapid rise of AI, machine learning methods for overcoming such challenges are advancing as well.
This symposium addresses challenges in video tracking, pose estimation and behavior recognition in laboratory animals. It presents AI and computer vision developments of the past couple of years and promising advances in the near future, focusing on machine learning methods and implementations for behavioral researchers.
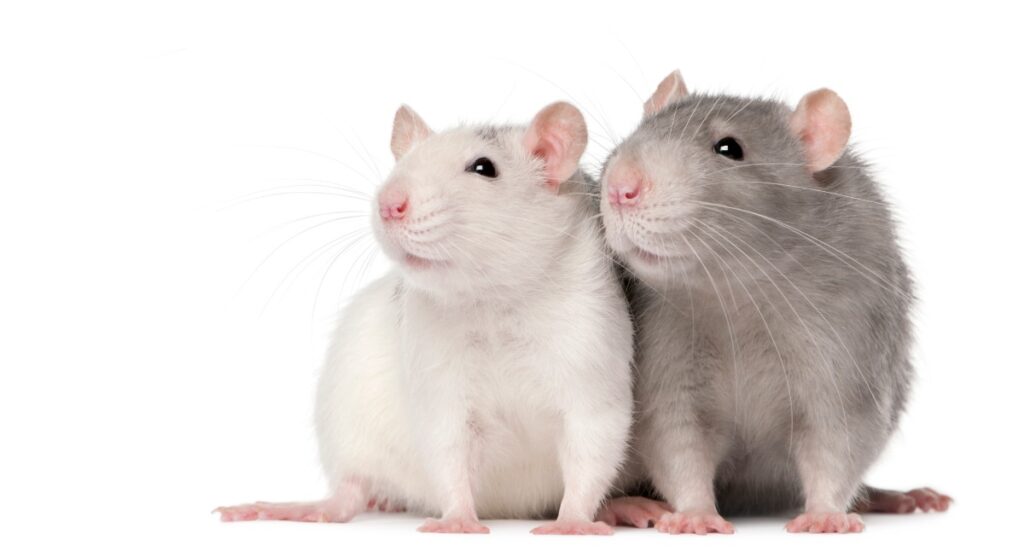